Statistical testing in non-interventional studies (NIS)
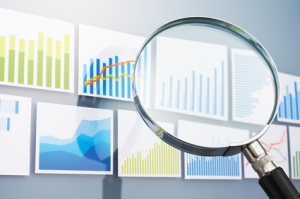
Statistical testing of pre-defined hypotheses is the most important methodology for analysis in comparative clinical trials. With respect to the investigated product, superiority tests versus placebo and non-inferiority tests in comparison with a reference medication are the most common applications.
In contrast, analysis of non-interventional studies (NIS) is mostly carried out by means of descriptive statistical methods (e.g. mean, standard deviation, frequency distributions). Unfairly, descriptive statistics are therefore often considered as “no real statistics” as the results of these methods are not based on p-values.
In this article we would like to address the question whether it is useful to perform statistical testing in the context of a NIS, as it is often requested by the sponsor, and why, if tests are performed, we should handle their results with caution.
The following example should help to understand the point.
Example
Let us consider an observational study in patients who were recently diagnosed with hypertension and subsequently started treatment with medication A.
Blood pressure was assessed at the start of the observation period and at the final visit after approximately 6 weeks. The data showed a mean decrease of 1 mmHg for systolic blood pressure (SBP) from 145 mmHg to 144 mmHg. The standard deviation of the changes was 7 mmHg.
Without any doubt, we can all agree that this small decrease of 1 mmHg in SBP cannot be considered as clinically relevant.
But is the result statistically significant?
The answer is: There is no absolute yes or no. It actually depends on the sample size.
To illustrate this, the table shows the p-values of a two-sided one-sample t-test, subject to sample size.
For this calculation we assume that the mean (1 mmHg) and the standard deviation (7 mmHg) of the decrease in SBP are the same for all sample sizes.
The table shows that the p-values decrease dramatically with increasing sample size, although the mean decrease (i.e. 1 mmHg) remains the same.
For N=200 patients we achieve a p-value < 0.05, which corresponds to statistical significance on the 5% significance level. The sample size for achieving p < 0.01 lies somewhere between 300 and 400.
Sample size | p-value |
---|---|
10 | 0.6621 |
50 | 0.3174 |
100 | 0.1563 |
200 | 0.0447 |
300 | 0.0139 |
400 | 0.0045 |
500 | 0.0015 |
600 | 0.0005 |
1000 | < 0.0001 |
What do we learn from this?
If the sample size is large enough, even the smallest effect may become statistically significant. The same applies for other statistical tests, e.g. for testing between two groups of patients (e.g. old vs. young, male vs. female).
That is why, in observational studies with a very large number of patients, where statistical testing has been requested, most or all of the p-values are < 0.05 or even < 0.001. Statistical significance however does not necessarily imply that the underlying effect is clinically relevant, which is obviously not the case in the above example.
By the way: If, in the context of a randomized clinical trial, the sample size is so large that even very small effects become statistically significant, we would refer to the study as being “overpowered“.
Consequences
The conclusion to be drawn is not that statistical tests in non-interventional studies should generally be avoided. They may be applied, but they must be interpreted with care.
Results of statistical testing should always be considered in relation to the clinical relevance of the findings, as exhibited by the descriptive analysis results – especially in NIS with large sample sizes.
Picture: @tadamichi /Fotolia.com