Artificial Intelligence (AI) in clinical research: transformation of clinical trials and status quo of regulations
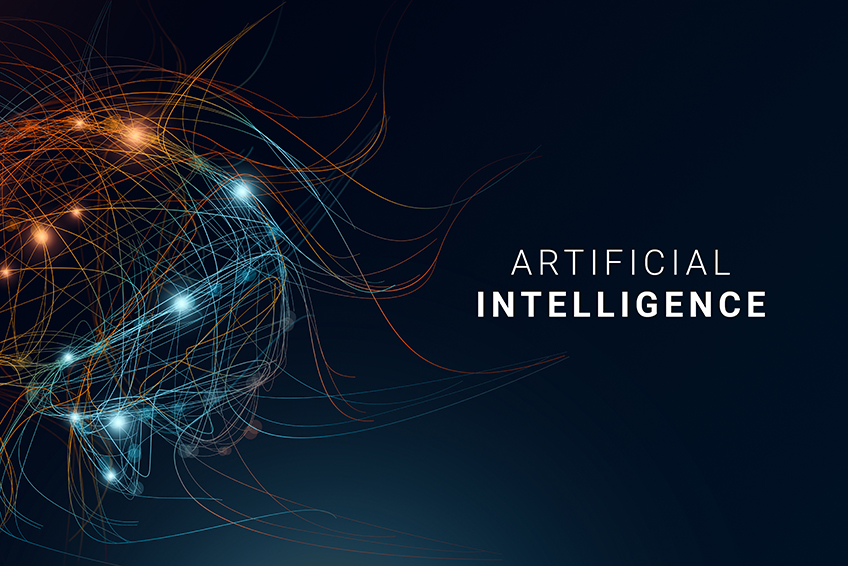
Artificial Intelligence (AI) supported technologies play a crucial role in clinical research: For example, during the COVID-19 pandemic the Biotech Company BenevolentAI found through a machine-learning approach that the kinase inhibitor Baricitinib, commonly used to treat arthritis, could also improve COVID-19 outcomes. The drug received authorization for emergency use by the FDA in 2021 (1).
Different industries increasingly use AI throughout the full drug discovery process as shown in the following use cases:
Research and discovery:
AI and machine learning support identifying optimal drug candidates. The Oxford-based Pharmatech Company Exscientia created in collaboration with pharmaceutical companies three drug candidates through AI technologies that entered Phase I clinical trials. This innovative approach allows for drug discovery in a significant shorter time compared to conventional research techniques (e.g. exploration research phase of the serotonin 5-HT1A receptor agonist DSP-1181 of less than one year) (2).
Another example for AI assisted research is Insilico Medicine, a biotechnology company that combines genomics, big data analysis and deep learning for in silico drug discovery. With its technology, Insilico Medicine discovered a molecule designed to inhibit the formation of substances that alter lung tissue in just 46 days (3). The drug candidate moved into trial phase in late 2021.
Preclinical phase:
The Directive on the Community code relating to medicinal products for human use (Directive 2001/83/EC, Annex I, Part 3, II A.1) foresees that in vivo experiments mustn´t be replaced (4). However, complimentary evidence is conceivable.
Therefore, AI-enabled technologies nowadays provide support in generating evidence to avoid redundancies at this stage. For example, the mentioned drug repurposing of Baricitinib to treat COVID-19 patients, discovered by AI-tools, allowed for building on existing evidence.
The German Federal Ministry of Food and Agriculture awarded two scientists with the 2021 Animal Welfare Research Prize for developing an automated manufacturing process of midbrain organoids. Organoids are an artificially grown mass of cells or tissue that resembles an organ. Drug candidates that prove to be ineffective or toxic to organoids may not require further testing in animal experiments. The combination of research with organoids at large scale with AI-based-analysis may yield even further potential of accelerating evidence generation during the preclinical phase (5).
Clinical development:
AI-enabled technologies may enhance operational efficiencies such as site and patient recruitment. For instance, IBM Health’s Watson for Clinical Trial Matching aims to collect and link structured and unstructured data from Electronic Health Records (EHR), medical literature, trial information and eligibility criteria from public databases (6)
AI-supported business intelligence platforms like GlobalData provide insights to identify sites with access to patient populations (7).
Another example is the platform Antidote that uses machine learning to match patients as potential participants with clinical trials (8).
Lastly, the pharmaceutical industry works on synthetic virtual control arms, meaning that the comparator group is modelled using real-world data that has previously been collected from sources such as EHR. The pharmaceutical company Roche already applied such an AI-driven model in a Phase II study (9).
Pharmacovigilance:
Pharmaceutical companies increasingly explore AI-enabled technologies that may support in pattern recognition and segmentation of adverse events (e.g. monitor conversations on social media and other platforms) (10). Furthermore, such technologies may automate manual processing tasks (e.g. translate and digitize safety case processing documents) (11).
How does the use of AI transform clinical research?
Change in the design and conduct of clinical trials
Usually it may take up to 12 years from discovery to marketing with involved costs of up to 2.6 billion US-Dollars. Therefore, AI support goes along with significant time and cost savings. For example, Insilico Medicine states that the process of discovering and moving its candidate into trial phase cost 2.6 million US-Dollars, significantly less than it had cost without using AI-enabled technologies (12).
Acceleration of patient recruitment and enrolment
The global Contract Research Organization IQVIA states that using machine-learning tools globally increased enrolment rates by 20.6 % in the field of oncology compared to traditional approaches (11). Furthermore, the early use of Watson for CTM led to an enrolment increase of 80 % in the 11 months after implementation (6).
Significant impact on orphan drug development
AI-enabled technologies might make specifically the usually cost-intensive Orphan Drug development more economically viable. However, data availability – also a common challenge in Orphan Drug trials – will be essential in this context.
Interdisciplinary collaborations are essential
Collaborations and networks across different sectors and industries will be key to ensure that AI fosters clinical research and has a positive impact on patient´s lives. Even additional research fields may emerge, as it is the case with Oculomics. Oculomics uses the convergence of multimodal imaging techniques and large-scale data sets to characterize macroscopic, microscopic, and molecular ophthalmic features associated with health and disease (13). Next to disciplines like sciences, information technologies and law, other expertise will gain importance like ethics and social sciences.
What about regulations?
So far, no harmonized regulatory framework exists for the use of AI in healthcare research. However, on cross-sectoral level the European Commission (EC) published within the Artificial Intelligence Act (AIA) a proposal of harmonized rules on Artificial Intelligence. It aims to “ensure that AI is safe, lawful and in line with EU fundamental rights” and therefore “stimulate the uptake of trustworthy AI in the EU economy” (14).
The AIA follows a risk-based approach. This means that high-risk AI systems (amongst others defined as systems that “pose significant risks to the health and safety or fundamental rights of persons” and systems that can “lead to biased results and entail discriminatory results”, ibid. Articles 32-40) will have to comply with mandatory requirements for trustworthy AI and undergo a conformity assessment. The conformity assessment is defined in the AIA and highlights specifically medical devices and in vitro diagnostic medical devices (ibid. Articles 30, 43).
Furthermore, the AIA addresses amongst others the prohibited uses of AI, obligations of providers and users, transparency requirements, regulatory sandboxes and expert laboratories, and penalties. After feedback iterations throughout the past years, the AIA is currently under review at the European Parliament.
The Committee on the Environment, Public Health and Food Safety released a position paper in April 2022 with three main concerns to be addressed:
- The risk of lacking consistency and standards in terms of regulatory approaches;
- The insufficient protection of the environment;
- The need to address not only users but also end recipients (15).
Currently the AIA is under review at the Committee on the Internal Market and Consumer Protection and the Committee on Civil Liberties, Justice and Home Affairs. The next step, planned by the end of September 2022, is for the European Parliament and the member states to adopt the Commission’s proposal and undergo the legislative procedure. While several interest groups commented publicly on the AIA and provided extensive position papers (e.g. Medtech Europe) clinical research representatives remain silent. The AIA addresses all sectors and does not specifically mention the area of clinical development. Therefore, specific implications in the field of clinical research may require an assessment on a case-by-case basis.
In conclusion, the areas of application of AI-enabled technologies and machine learning in clinical research are manifold and pull through the full drug discovery process. As shown in the use cases AI-enabled technologies and machine learning facilitate significant breakthroughs in clinical research. As with other industries, this is the beginning of an unknown road with respective regulations still in its very infancy. With the AIA the EC introduced a first attempt to regulate the application of AI on cross-sectoral level to ensure compliance with fundamental rights. It remains to be seen how this will impact the use and development of AI-enabled technologies in the field of clinical research.
References
[1] https://www.benevolent.com/covid-19
Accessed May 19, 2022
[2] https://www.exscientia.ai/
Accessed May 19, 2022
[3] Zhavoronkov, A., Ivanenkov, Y. A., Aliper, A., Veselov, M. S., Aladinskiy, V. A., Aladinskaya, A. V., … & Aspuru-Guzik, A. (2019).
Deep learning enables rapid identification of potent DDR1 kinase inhibitors.
Nature biotechnology, 37(9), 1038-1040.
[4] https://eur-lex.europa.eu/LexUriServ/LexUriServ.do?uri=CELEX:32001L0083:EN:HTML
Accessed May 19, 2022
[5] Renner, H., Schöler, H. R., & Bruder, J. M. (2021).
Combining Automated Organoid Workflows with Artificial Intelligence‐Based Analyses: Opportunities to Build a New Generation of Interdisciplinary High‐Throughput Screens for Parkinson’s Disease and Beyond.
Movement Disorders, 36(12), 2745-2762.
[6] https://www2.deloitte.com/content/dam/insights/us/articles/22934_intelligent-clinical-trials/DI_Intelligent-clinical-trials.pdf
Accessed May 19, 2022
[7] https://www.globaldata.com/
Accessed May 19, 2022
[8] https://www.antidote.me
Accessed May 19, 2022
[9] Davies, J., Martinec, M., Delmar, P., Coudert, M., Bordogna, W., Golding, S., … & Crane, G. (2018).
Comparative effectiveness from a single-arm trial and real-world data: alectinib versus ceritinib.
Journal of comparative effectiveness research, 7(09), 855-865.
[10] https://www.pfizer.com/news/articles/ai-drug-safety-building-elusive-%E2%80%98loch-ness-monster%E2%80%99-reporting-tools
Accessed May 19, 2022
[11] https://www.iqvia.com/-/media/iqvia/pdfs/library/white-papers/ai-in-clinical-development.pdf
Accessed May 19, 2022
[12] https://www.handelsblatt.com/technik/medizin/neue-medikamente-pharmaindustrie-nutzt-kuenstliche-intelligenz-zur-arzneimittelforschung/28161478.html
Accessed May 19, 2022
[13] Wagner, S. K., Fu, D. J., Faes, L., Liu, X., Huemer, J., Khalid, H., … & Keane, P. A. (2020).
Insights into systemic disease through retinal imaging-based oculomics.
Translational vision science & technology 9(2), 6-6.
[14] https://artificialintelligenceact.eu/the-act/
Accessed May 19, 2022
[15] https://www.europarl.europa.eu/doceo/document/ENVI-AD-699056_EN.pdf
Accessed May 19, 2022
Picture: @Prostock-studio/AdobeStock.com